Background
Clustering is an unsupervised machine learning approach where the aim i to determine “natural” or “data-driven” groups in the data without using apriori knowledge about labels or categories. The challenges in unsupervised clustering is that it always produces a partitioning of the samples since each clustering method implicitly impose a structure on the data. The question is: What is a “good” clustering? We need to evaluate the results based on the clustering tendency, number of clusters and the clustering quality.
Aim
clusteval
is a Python package that is developed to evaluate the clustering tendency, number of clusters and clustering quality. clusteval
returns the cluster labels for the optimal number of cluster that produces the best partitioning of the samples. The following evaluation strategies are implemented: silhouette, dbindex, and derivative which can be used in combination with agglomerative and kmeans clustering. In addition dbscan and hdbscan is implemented for which an internal gridsearch scheme will determine the best partitioning.
Note
The clusteval
library gridsearches across the number of clusters, and method-parameters to determine the optimal number of clusters given the input dataset.
Quickstart
A quick example how to learn a model on a given dataset.
# Import library
from clusteval import clusteval
# Initialize
cl = clusteval()
# Generate random data
X, y = cl.import_example(data='blobs')
# Fit data X
results = ce.fit(X)
# Plot
ce.plot()
ce.plot_silhouette()
ce.scatter()
ce.dendrogram()
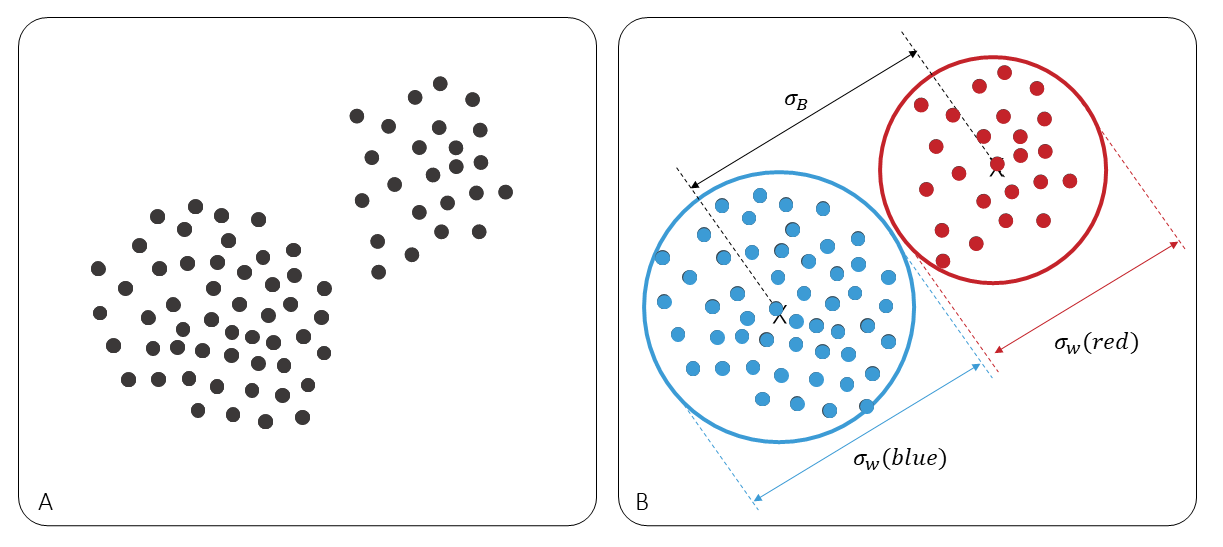